Knowledge
The Knowledge Category
Our knowledge is the body of facts and information with which we are aware or familiar. It’s the first category of data literacy traits enumerated because our development starts with the knowledge that we obtain either through academic study or through practical experience.
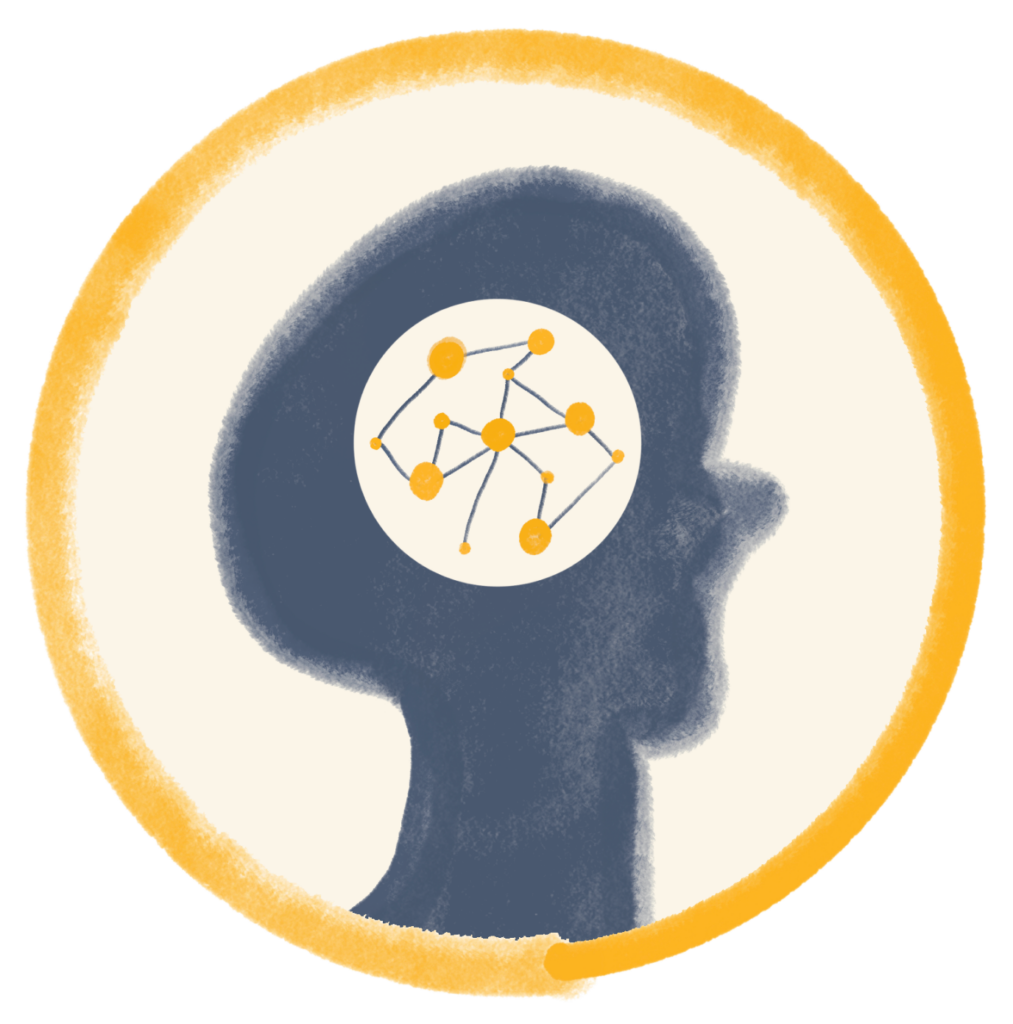
So, what does a data literate person know? A data literate person knows, among other concepts…
- Basic Elements of Data
- Data Storage Methods
- Data Analysis Principles
- Data Visualization Rules of Thumb
- AI Systems & Capabilities
Knowledge Category Quote
The quote for the Knowledge category was provided by Alberto Cairo, Knight Chair at the University of Miami, and author of “How Charts Lie” (2019) and “The Truthful Art” (2016).
Note Alberto’s emphasis on the intuitive and tentative nature of the knowledge we can acquire about data.
Knowledge Category Traits
1. Basic Elements of Data
Understanding of both traditional data types (categorical, quantitative) and unstructured data types (text, images, audio, video), including how these serve as building blocks for both statistical analysis and AI model training
The data and AI literate person knows how to distinguish between different types of data, from traditional structured types such as categorical and quantitative variables, to unstructured data like text, images, audio, and video. Beyond merely identifying data types, they understand what can and can’t be done with them in both traditional analysis and AI applications.
Just as the poet studies vowels and consonants, verbs and nouns, individuals who are highly literate in data and AI embrace the fundamental building blocks of both structured and unstructured data. This enables them to see data for what it is – information that can be grouped, aggregated, summarized, and processed by both human and machine intelligence to generate powerful insights and messages.
Learning Resources
- Course: Data Literacy Fundamentals
- Video: Data Literacy Whiteboard 001 – Four Data Scale Types (Apr 19, 2019)
- Paper: JSTOR: “On the Theory of Scales of Measurement” by S.S. Stevens (June 7, 1946)
2. Data Storage Methods
Familiarity with how data is collected, structured, and stored across various systems, from spreadsheets and databases to modern data lakes and AI-ready architectures
The person who is highly literate in data and AI is familiar with ways that data is collected, structured, and stored, and the attributes associated with each approach. They understand traditional storage systems like spreadsheets with their cells in rows and columns, databases with their records arranged in relational tables, as well as modern data architectures that support AI operations, including data lakes and model storage systems.
Similar to how farmers are knowledgeable about growing, harvesting, and storing grain in silos, people who are highly literate in data and AI understand various ways that data is collected, structured, and archived. They’re aware of different ways data is imported, exported, and transformed between various storage types, including how data needs to be organized and versioned for AI model training and deployment.
Learning Resources
- Blog post: “Spreadsheet Thinking vs. Database Thinking” by Robert Kosara (April 24, 2016)
- Article: Database basics | Microsoft Support
- Article: What is a cloud database? | IBM Think Topics
3. Data Analysis Principles
Understanding of fundamental statistical concepts and AI/ML principles, including the comparative strengths of traditional versus AI approaches and factors affecting their reliability
Those who are highly literate in data and AI understand that storing data is not an end in itself, but rather a means of extracting valuable insight about one’s environment. They grasp the fundamental principles of both traditional statistical analysis and modern AI/ML concepts, understanding when each approach is most appropriate.
Much like an investigator knows what types of deductive and inductive reasoning are useful when solving a crime, a person who is highly literate in data and AI appreciates critical thinking, statistical significance, and what types of insights can be reasonably derived from both traditional analysis and AI-powered approaches. They understand the strengths and limitations of each method, knowing when to apply classical statistics versus when to leverage AI capabilities.
Learning Resources
- Course: Data Literacy Level 2 – Working Effectively with Data
- Paper: “Elements and Principles of Data Analysis” by Stephanie C. Hicks & Roger Peng (March 2019)
- Book: The Art of Statistics: How to Learn from Data (David Spiegelhalter, 2021)
4. Data Visualization Rules of Thumb
Knowledge of how humans perceive and interpret visual information, including principles related to different kinds of charts, graphs, and maps, along with the display of uncertainty and AI model outputs
Since, according to Dr. Tamara Munzner, the human visual system is a “very high bandwidth channel to the brain,” the person who is highly literate in data and AI understands various ways to visualize both traditional data analysis and AI model outputs. They grasp the principles of cognition relating to how humans decode visual encodings such as position, length, area, and color, and understand how to effectively represent AI-specific concepts like model uncertainty and decision processes.
Like a doctor who knows how the body will react to prescribed medications and therapies, those who have high levels of data and AI literacy know how their audience members will interpret various visual treatments and forms. They understand how to create visualizations that make both traditional analysis and AI-driven insights clear and accessible to their intended audience.
Learning Resources
- Course: Data Literacy Level 1 – Learning to See Data
- Book: Visualization Analysis & Design (Tamara Munzner, 2014)
- Lesson: Data Types, Graphical Marks, and Visual Encoding Channels by Jeffrey Heer, Observable (May 15, 2024)
5. AI Systems & Capabilities
Understanding of major AI system types, their capabilities and limitations, including knowledge of when AI can reliably augment human decision-making and when traditional analytical approaches may be more appropriate
The person who is highly literate in data and AI understands the landscape of AI systems, their capabilities, and their limitations. They know the major types of AI applications, from language models to computer vision systems, and understand when these tools can reliably augment human decision-making versus when traditional analytical approaches may be more appropriate.
Like a mechanic who knows which tools to use for different repairs, they understand the strengths and limitations of various AI systems. They recognize that AI tools, while powerful, are not magical solutions but rather sophisticated information processing systems that require appropriate data, careful implementation, and human oversight to be effective.
Learning Resources
- Course: AI Literacy Fundamentals – Helping You Join the AI Conversation
- Book: Artificial Intelligence: A Guide for Thinking Humans by Melanie Mitchell
- Video: Overview Artificial Intelligence Course | Stanford CS221: Learn AI (Autumn 2019)
Self-Assessment: Knowledge Category
Use the self-assessment worksheet and scoring guide that you downloaded in the Introduction lesson to score yourself in proficiency and importance for each of these five traits in the Knowledge category, giving yourself between 0 and 4 points for each score.
Hypothetical Case Study: “The Healthcare Analytics Transformation”
The Situation:
A regional healthcare system was struggling to make sense of their patient data and newly implemented AI diagnostic tools. Priya, a clinical operations manager with strong data and AI literacy, was tasked with helping departments better understand and use their data resources.
The Challenge:
The organization had:
- Uncertainty about when to use AI versus traditional analysis
- Multiple data sources (electronic health records, imaging data, patient feedback)
- New AI diagnostic tools that many staff didn’t understan
- Confusion about data types and storage methods
Actions Taken:
Priya demonstrated strong knowledge traits by:
- Basic Elements of Data: Helping staff understand different types of patient data (categorical demographic data, continuous vital signs, unstructured physician notes, imaging data)
- Data Storage Methods: Mapping out how different data types were stored across systems and implementing a clear data architecture
- Data Analysis Principles: Creating guidelines for when to use traditional statistical analysis versus AI tools for different diagnostic scenarios
- Data Visualization Rules of Thumb: Developing standardized visualization approaches for different types of patient data
- AI Systems & Capabilities: Clearly explaining the strengths and limitations of their AI diagnostic tools
Results:
- Staff became more confident in understanding and using different types of data
- Clear protocols were established for using AI diagnostic tools
- Department efficiency improved by 30%
- Medical staff reported better understanding of when to rely on AI versus traditional methods
Key Takeaways:
This case demonstrates how strong knowledge traits enable someone to:
- Maintain appropriate balance between traditional and AI-powered approaches
- Bridge the gap between technical and non-technical stakeholders
- Create clear frameworks for data and AI usage
- Build organizational confidence in new tools